图像直方图
直方图均衡化
https://zhuanlan.zhihu.com/p/44918476
假如图像的灰度分布不均匀,其灰度分布集中在较窄的范围内,使图像的细节不够清晰,对比度较低。直方图均衡化,对图像进行非线性拉伸,是一种灰度的变换过程,将当前的灰度分布通过一个变换函数,变换为范围更宽、灰度分布更均匀的图像。这样,原来直方图中间的峰值部分对比度得到增强,而两侧的谷底部分对比度降低,输出图像的直方图是一个较为平坦的直方图。
原理
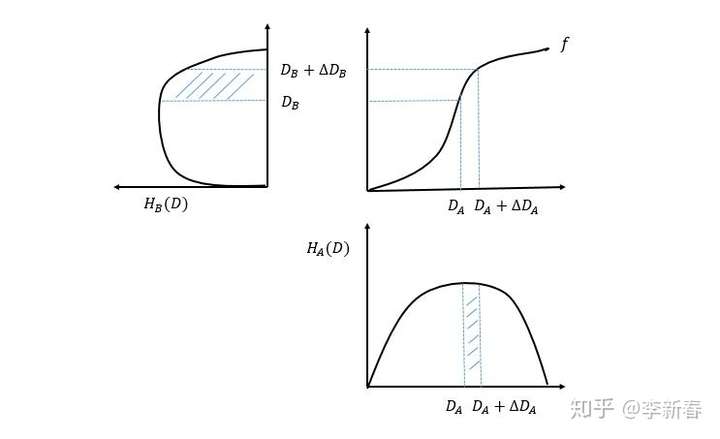

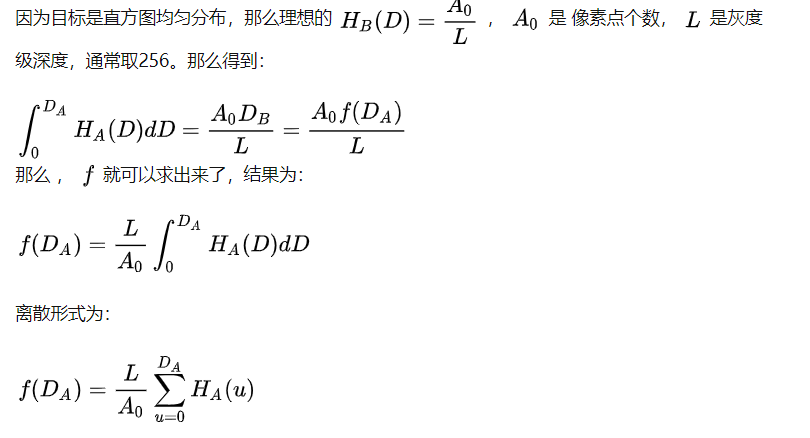
计算步骤
- 计算原始图像的直方图
- 计算累计直方图 CDF
- 映射为 256 /(m x n ) x CDF
1 2 3 4 5 6 7 8 9 10
| hists = histogram(img)
hists_cumsum = np.cumsum(hists) const_a = level / (m * n) hists_cdf = (const_a * hists_cumsum).astype("uint8")
img_eq = hists_cdf[img]
|
直方图匹配
https://blog.csdn.net/qq_31347869/article/details/89514253
如果希望得到具有规定形状的直方图,就需要用到一种特殊的处理方法:直方图匹配 (直方图规定化)。原理很简单,有了参考图,可以计算它到规范化后的变换 ,同时也可以计算 低光图到 规范化后的变换。r -> s -> z s是中间规范化后的像素值 。最后求逆映射的时候不用显式的求,找最近的即可。
1 2 3 4 5 6 7 8 9 10 11 12 13 14 15 16 17 18 19 20 21 22 23 24
| import cv2 import numpy as np import matplotlib.pyplot as plt img = cv2.imread('C:\\Users\\admin\\Desktop\\original_img3\\testimg\\lena_300_500.jpg') ref = cv2.imread('C:\\Users\\admin\\Desktop\\original_img3\\testimg\\messi_300_500.jpg') out = np.zeros_like(img) _, _, colorChannel = img.shape for i in range(colorChannel): print(i) hist_img, _ = np.histogram(img[:, :, i], 256) hist_ref, _ = np.histogram(ref[:, :, i], 256) cdf_img = np.cumsum(hist_img) cdf_ref = np.cumsum(hist_ref) for j in range(256): tmp = abs(cdf_img[j] - cdf_ref) tmp = tmp.tolist() idx = tmp.index(min(tmp)) out[:, :, i][img[:, :, i] == j] = idx cv2.imwrite('C:\\Users\\admin\\Desktop\\lena.jpg', out) print('Done')
|
局部对比度增强
就是将全局直方图均衡的思想应用于邻域直方图处理中